Preliminary AI puzzle model predict protein complex structure
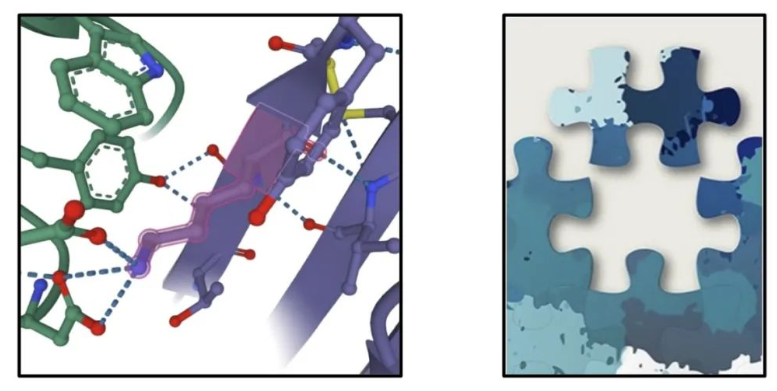
The molecules stated that learning plays a vital role in the study of AI auxiliary drugs. In traditional drug development, the commonly used molecular docking models need to perform a large number of configuration sampling and optimization, and screen a relatively stable structure. Such strategies are relatively low -efficiency and are difficult to apply to high -throughput protein docking tasks. The Harmonic Molecular Reprerentation (HMR) described in this article has achieved more accurate and efficient protein docking model development. HMR uses the two -dimensional Riman streaming modeling molecular surface to combine the combination of analytical analysis technology and neural network to achieve the comparison of the multi -scale transmission of multi -scale propagation, the multi -scale communication of the chemical signal, and the matching degree of the two protein surfaces. Rigid Protein Docking (Rigid Protein Docking). Experiments show that the HMR -based molecular docking model has higher accuracy than the current deep learning SOTA [1], and the traditional molecular docking method is accelerated by more than 100 times. Related papers have been included in ICLR 2023.
The interaction between protein is an important foundation for realizing its biological activity. For example, the human body can produce antibody protein (the green part above the figure) and the invasive germs (purple parts) to inhibit the disease. Biopharmaceutical research analyzes the physical and chemical mechanisms of interaction between biomolecules, and further designs new types of drug molecules (such as developing new crown antibodies) that can be combined with some specific targets. In the micro scale, the combination of protein is mainly determined by the force between molecular, such as hydrogen bonds, static electricity, and hydrophobic effects.
In traditional drug development, molecular docking technology (Molecular Docking) models the stable structure of the interaction of two molecules in the real creature through physical methods. These traditional molecular docking models need to perform a large number of configuration sampling and optimization, and screen out a more stable structure as the prediction result. This strategy based on sampling and screening has caused the traditional method to be more efficient and difficult to apply to a protein docking task that is difficult to apply to high -throughput. To. The accurate and efficient molecular docking model can help quickly screen protein molecules suitable for wet experiment testing, thereby improving the efficiency of new drug research and development.
In order to develop more accurate and efficient protein docking models, byte ByTedance Research team has designed a set of geometric deep learning solutions based on molecular surfaces. The core idea of this scheme is to understand the interaction between protein from the perspective of the puzzle, and to achieve the protein complex configuration prediction according to the idea of the puzzle.
Specifically, if the two proteins can be combined, the combined area must meet the two conditions: chemical matching and complementary geometric structure. Therefore, an analogy of analogy can be intuitive: as long as the “puzzle” that can be matched with two shapes and textures can be found on the protein surface, then these two proteins can be spelled together to form a stable protein complex.
Based on the above assumptions, the team proposed a Harmonic Molecular Representation (HMR) based on the molecular surface of Riemann: use the two -dimensional Riemann -shaped modeling molecular surface to combine the combination of analytical analysis technology and neural network to realize the upper geometric geometric geometric geometry Comparison of the multi -scale communication of chemical signals and the matching degree between the two protein surfaces, and then use the logic of the “protein puzzle” to achieve the protein molecular rigidity connection (Rigid Protein Docking). In intuitive, neural network models need to study geometry and chemical laws of puzzles from the structure (training set) of a large amount of protein complex, so as to predict that the protein complex structure that has not been seen before can be predicted.
Experiments show that the HMR -based molecular docking model has higher accuracy than the current deep learning SOTA [1], and the traditional molecular docking method is accelerated by more than 100 times. The next three chapters will introduce the main ideas and technical summary of Riemann’s streaming molecules, molecular surface geometric deep learning, and protein puzzle models.
https://oaicon.com/index.php/2023/03/05/preliminary-ai-puzzle-model-predict-protein-complex-structure/
The surface of the biomolecular molecule usually refers to the interface formed by the molecule in the solution with the solvent (such as water molecules). We can express this interface as a two -dimensional Riemannian Manifold in a three -dimensional space. The figure above shows the surface streaming structure of an antibody protein under different resolution, and the static potential function distribution on the corresponding molecular surface on the flow of the current. In other words, the structure of the streamlined structure outlines the shape of the molecule, and the function distributed on the current can represent the chemical properties of the molecular surface. Therefore, the use of Riemann can uniformly represent the geometric structure and chemical properties of the molecule, thereby integrating molecular information and helping the AI model to better learn the structure of protein -active relationship.
Under Riemann’s streaming, each molecule has a unique set of “Shape-DNA”. These shape genes are defined as the collection of Laplace-Beltrami Operator (LBO), which is defined as the molecular surface-shaped Laplaz-Beltrami Operator (LBO). Methods are not affected by the position and orientation of the molecules in the three -dimensional space.
The LBO of these shape genes {φᵢ} constitutes a group of (standard orthodontics) base functions on the molecular surface flow. We can simply expand the Fourier group composed of their analogies into sine / Yu String functions on Riemann. Therefore, these shape genes and base functions can help us conduct harmonic analysis on the molecular surface, that is, the form of a linear combination of a series of base functions (below). For the surface of the same molecular surface, different chemical properties (such as hydrophobic and static potential energy) can be very concisely represented as a set of linear combination coefficients (one -dimensional array [C₀, C₁, C₂, …]).
This Riemann streaming is a direct modeling of the molecular surface. The obtained shape genes and the LBO base function are affected by different discrete modeling methods. Method [2]), therefore increase the robustness of modeling.
§2 Molecular surface geometric depth learning
The previous chapter mainly introduces how the article uses the geometric structure of the Riemann -shaped modeling molecular surface (corresponding to the shape of the puzzle). This chapter briefly describes how to train the chemical properties of the neural network learning molecular surface (corresponding to the texture on the puzzle).
Using the shape genes and LBO base functions of the molecular surface to model the geometric and chemical properties of modeling molecule provided us with a new molecular representation of learning ideas. The surface -based molecular modeling focuses on the description of the external characteristics of the molecularity. It may be more advantageous than that of the downstream task (such as protein interaction) than the three -dimensional graphic neural network (Euclidean Graph Neural Networks) based on amino acids or all atomic modeling. “Shape gene” is a modeling of different frequencies (or particle size) signals on the overall surface of the molecular surface. It does not need to pre-cut the surface area in advance [2], which also allows the model to be suitable for the molecular information of different standards (Multi-SCALE) Essence
Based on this set of ideas, the team designed a set of geometric deep learning schemes for global information transmission on the molecular surface, thereby helping the neural network to learn more geometric and chemical properties of molecular surface. The article proposes a new method of Manifold Harmonic MESSAGE PASSING: a kind of current information transmission mechanism similar to the heat diffusion, but the modeling is more flexible. The thermal diffusion mechanism can be regarded as a low -pass filtering operation of the signal, so low -frequency signals are easier to spread than high -frequency signals [3]; while the flow adjustment information transmission allows the band of different frequencies Independent propagation [4], so the communication distance is farther, indicating that the particle size is thinner. Combined with the neural network structure such as residual connections, the method proposed in the article can learn the geometric and chemical information of different scale and different distances on the surface of the molecular surface, so as to have better modeling capabilities for molecules.
§3 Protein puzzle AI model
Now, we have (§1) the two tools of molecular surface chemical function learned by the Riemann -based molecular geometry and (§2) neural network. Prediction.
Specifically, the structure of the protein and ligand protein is given, and we hope to predict the configuration of them in combination. Here are two sub -problems: (1) Where are the binding sites; (2) what kind of space posture of the receptor -ligaine. The article proposes that the binding site between protein should have two important conditions: complementary geometric structure and chemical matching, so the predictive predict of protein complex configuration can be regarded as a “protein puzzle” problem. Similar to the idea of human solution to puzzle: First find the stitching surface (predictive binding site) between two puzzles, and then use the similarity of the shape and pattern to rotate one of the puzzles to the correct position (molecular docking).
In terms of specific model construction, the team first uses the HMR module proposed in the text to perform Binding Site Prediction. The characteristic learning of the molecular surface is implemented through a flow information transmission mechanism, and information communication between protein molecules is implemented to introduce the characteristics of the molecular surface and introduce the cross -attention mechanism. The final output of the module is whether a model of the model is a binary prediction of a protein binding site on a certain area on the surface of the molecular surface. It can be understood that this step corresponds to a piece of lack of lack of puzzles.
Next, enter the molecular docking module: The predicted protein binding should have a certain function correspondence on the surface, because the interaction between molecules is determined by matching chemical (such as static power and hydrogen bond). In other words, the corresponding texture on the puzzle that can match should also be consistent. According to such assumptions, the team further uses the Functional Map to convert the corresponding relationship between the corresponding relationship into the corresponding relationship between the receptor-ligand binding site, and use the KABSCH algorithm to put the ligand molecules for space for space The rotation is shifted peacefully, and the protein complex structure after the docking is finally obtained.
This “protein puzzle” method and three -dimensional diagram neural network method Equidock [1] and traditional methods based on the “sampling -screening” strategy (ATTRACT and HDOCK). On the protein docking standard test set Docking Benchmark 5.5, the “protein puzzle” method has achieved better results in each measurement item than the three -dimensional diagram neural network, even close to some traditional methods. And this method of deep learning -based methods predicts the speed of molecular docking by more than 100 times compared with the traditional method.
§4 Summary
This work proposes a new way of deep learning modeling based on the molecular surface. It uses Riemann to model the properties of chemistry, physics, and geometric -related in Riemann. Figure deep learning method of neural network. In conclusion:
-Different from common modeling methods based on sequences or two -dimensional / three -dimensional map structures, the team uses surface -based molecular modeling methods. This modeling idea not only retains the overall three -dimensional structure of the molecule, but also avoids redundant modeling of the internal structure of the macromolecular. It may be more advantageous in surface tasks related to the surface of protein function and protein.
-Chirmani -forming directly models the molecular surface, and uses molecular shape genes and corresponding functions to represent the function distribution of the surface. This modeling method is not affected by the surface discrete sampling and triangular section, so it has better robustness. The team also demonstrated the surface -to -profile deep learning module based on the concept of reconciling and filtering and filtering concepts, as well as the surface -to -be -connecting method based on pan -letter mapping.
-In the text, the idea of using this surface modeling method and “puzzle” realizes the deep learning model Mercy of protein rigid molecules. Compared to the current deep learning model based on the three -dimensional diagram neural network, it has achieved better results, and it has also greatly improved in terms of speed compared to traditional methods.