The four major trends of the Internet of Things analysis in 2023
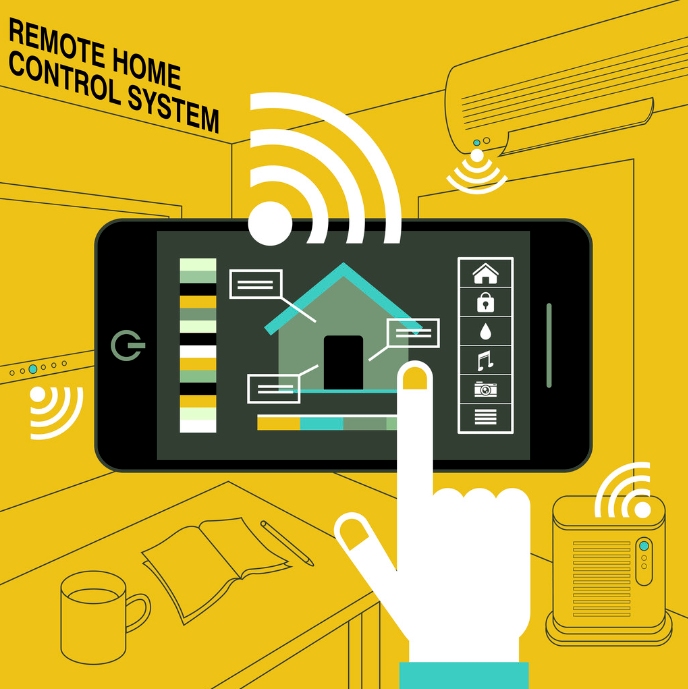
Over the years, analysis has become an indispensable part of the Internet of Things. Industrial organizations such as manufacturers, transportation and energy companies, and governments around the world continue to adopt these technologies to improve operating efficiency and achieve significant cost and operational conservation.
Advanced analysis technologies such as artificial intelligence, streaming analysis and machine learning are combined with the Internet of Things technology and sensors, which can help smart factories, power grid infrastructure and even urban power supply. But what will happen to this important area in 2023?
The rise of IoT analysis
In the next year, there will be four major trends in IoT analysis: the rise of low -code and non -code automatic machine learning (AutoML), enhanced digital twin technology, industrial applications of computer vision, and the boundaries between edges and clouds. These trends do not mean that they are different from previous years, but the continuation of the market trajectory after the epidemic.
It is estimated that by 2023, through low code and unpaid code automl, industrialized artificial intelligence will have greater availability. These models are provided by the self -service market and may be enhanced by customization and deployment of packaging services.
In 2023, we will also see more special digital twin applications specifically for energy, infrastructure optimization and industrial manufacturing definition. It is expected that each organization will also use computer vision and other artificial intelligence technologies. Industries that use these technologies will be extended to more niche cases of IT personnel and data scientists. The computer vision plan will focus on increasing production and operations. Efficiency and security.
Finally, with Microsoft Azure, Amazon Network Service, and Google Cloud Platform, large -scale enterprises have begun to launch core cloud services on the edge. Edge computing will become an extension of cloud computing. Working load is intelligently distributed in a mixed environment. This will mean a faster IoT analysis in 2023 to enhance the decision -making at the source.
Low code, no code
We will continue to see the extensive adoption of various industries for the Internet of Things plan. The momentum in this field has continued for a long time. If we review three or four years ago, what we really pay attention to is the idea of concept verification, but now our customers are transitioning from these POCs to more sustainable and long -term concepts.
In the past year, we have seen some companies hope to test the Internet of Things and analyze projects and prove that they can continue to create value. This is not necessarily that we will see the transformation from last year to next year, but as the customers begin to see the significant return of their projects, from narrow POC to wider use.
It seems difficult to imagine that analysis is not an integral part of each Internet of Things. However, as the system around it becomes easier to understand and deploy widely, it has gradually changed in several years. The rise of low -code and no code analysis is the main driving force for accessability.
The biggest goal of low -code and non -code analysis is to allow anyone to convert data into insights. The low code and non -code environment are open to those companies without a lot of data scientists. One of the industry. Analysis and data are no longer the field of white -collar and blue -collar workers, and it has begun to be used by everyone in the supply chain.
Twin
The surge in sensors also means that the system becomes simpler and simpler in the digital environment, which will lead to the next predictive enhancement of digital twin technology trends.
Once we can accurately copy the real world system in the digital world, we can start using variables to optimize physical elements without affecting daily operations. Nowadays, you can also start creating digital twins for infrastructure, and start to move these leverage to predict whether there are problems in any part of the supply chain, and even measures to solve the problem before the problem occurs.
In the past, most analysis procedures involved a large amount of data, moved data through the network, and put them into the consistent environment. Then there is the process of creating algorithms. These algorithms can view data and generate insights, and then distribute them to consumers.
Seven or eight years ago, the application of analysis technology expanded to the Internet of Things. This is actually more about the expansion of the ecosystem, not a complete change. In the past, most of the analysis programs involved a large amount of data, moved data through the network, and put them into a consistent environment. Then there is the process of creating algorithms. These algorithms can view data and generate insights, and then distribute them to consumption. The changes in sensor technology reshaped the entire field. A cheaper and stronger sensor becomes widespread. Their deployment helps to bring decision -making to the source of the data. On the edge of the sensor, a strong analysis of real -time flow data is used.
Industrial application of computer vision
Many people think that computer vision is object detection. But this is an area where we see a lot of growth, which has a wide range of applications. We can use it to identify the areas that need to be monitored and set up alarm to warn what the operator happens. Over time, it is determined that they can correct the problems of the problem of correction.
Of course, a huge benefit of this technology is predictive maintenance, allowing operators to identify and solve areas that are particularly prone to accidents or problems. We often see more widely used applications, not just predictive maintenance. Usually it is a real -time operation defect detection.
The biggest advantage of computer vision is that it is usually not a displacement technology, and does not require a large number of sensors or changes to the system or device. It can be as simple as deploying cameras. This is a low -impact measure that can greatly improve the quality of predictive maintenance or safety.
The boundary between blurred margins and clouds
There was a clear boundary between internal deployment or cloud computing and edge computing. The edge is the field of network companies, and they provide distributed equipment located outside the cloud. In the past 12 to 18 months, as companies have closer to the sources of data, the marginal computing on the cloud infrastructure has accelerated the development of marginal analysis and the decision -making that generated..
https://oaicon.com/index.php/2023/03/08/the-four-major-trends-of-the-internet-of-things-analysis-in-2023/
The transformation from cloud computing to internal deployment has caused the emergence of a mixed environment. All the items we see have a consistent route, which helps to determine the scope of the problem and be able to target specific results. This is where we see that enterprises perform well in the use of analysis technology, not just machine learning or the Internet of Things. But I think this is one direction for everyone to achieve the greatest success in the shortest time.